Making sense of too much data
Ryan Noone
Jul 28, 2022
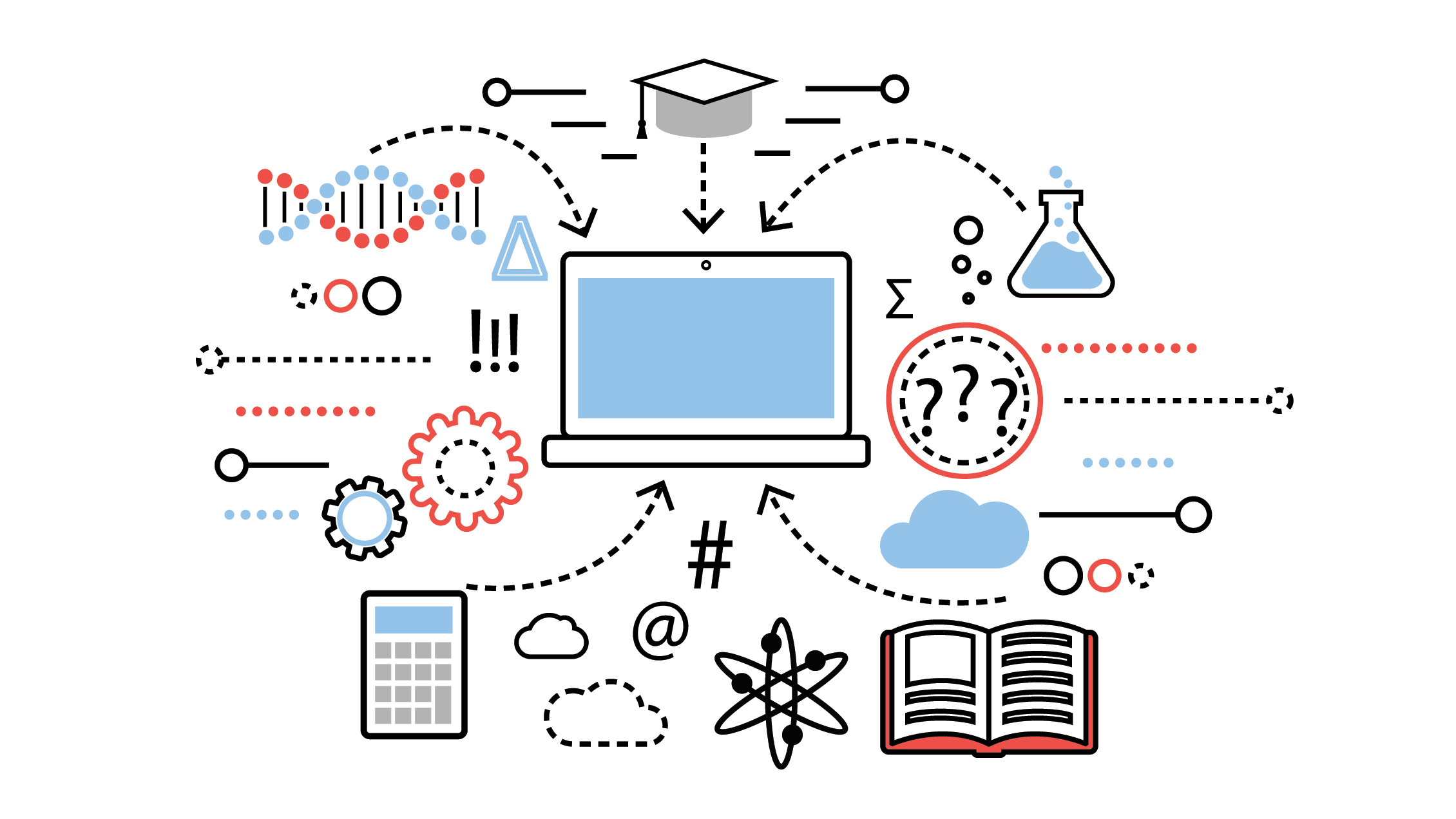
With hundreds of research papers published each day, synthesizing all of the available information for literature reviews has become increasingly difficult. Reading and extracting data from thousands of papers can be daunting and nearly impossible for the average-sized research group. To combat this issue, professors and librarians at Carnegie Mellon University are teaming up to find and teach unique techniques to uncover pertinent information for academic studies.
"There is a water hose of information, and it can become tough to figure out what's going to be valuable to the specific research you're working on," says Liz Wayne, assistant professor in CMU's Department of Chemical Engineering. "That can lead to challenges where you only hear from the biggest names in the field or focus only on the most recent publications."
Dr. Wayne's research focuses on nanoparticle target strategies for modulating macrophages in cancer therapy. Macrophages are large phagocytic cells found either in stationary form in human tissue or as mobile white blood cells and play an essential role in eliminating many diseases. Cancer is also a very complex topic that generates many different types of research. Searching for either of these "keywords" results in an endless amount of research to sift through, making it difficult to determine what's relevant to the study at hand.
So, Wayne began thinking of ways to narrow her search. First, she reached out to CMU Librarians Sarah Young and Melanie Gainey to help find and locate the many papers published on these topics.
"The library brings an expertise in the systematic searching of information," says Young. "We're trained on tools that allow us to discover and organize large amounts of literature on any given topic."
Next, she contacted her colleague, John Kitchin, a professor in CMU's Department of Chemical Engineering and an expert in machine learning and Artificial Intelligence (AI). Utilizing these instruments, Kitchin worked with Wayne to determine the type of information she was looking for, then began training algorithms to perform natural language processing, a component of AI that helps computers understand human language. This allowed the pair to quickly categorize all the information the library had found into "buckets," revealing what was relevant and what wasn't.
"Natural language processing works by turning the words in a document into vectors," says Kitchin. "These vectors are numerical and represent not only specific words but also the words around them, allowing the computer to categorize papers into groups, suggesting which should be included and excluded for consideration in a particular study."
After finding success by combining these methods, the uniquely skilled group of faculty members and librarians began discussing how to share their approach with students. Coming up with a curriculum and way to implement these ideas into the classroom, the group applied to the CMU Eberly Center's Innovative Models for Undergraduate Research Faculty Fellows Program (IMUR). The initiative is designed to support faculty in exploring and developing innovative models for undergraduate research and creative inquiry, allowing for the creation of new opportunities for student learning and engagement.
Now, in its first year, the course “Information Overload: Systematic Methods for Understanding What We Know” is providing students from various disciplines the opportunity to learn aspects of systematic searching and artificial intelligence to incorporate into their current and future research endeavors.