CMU becomes go-to place for machine learning in catalysis research
Adam Dove
Dec 3, 2019
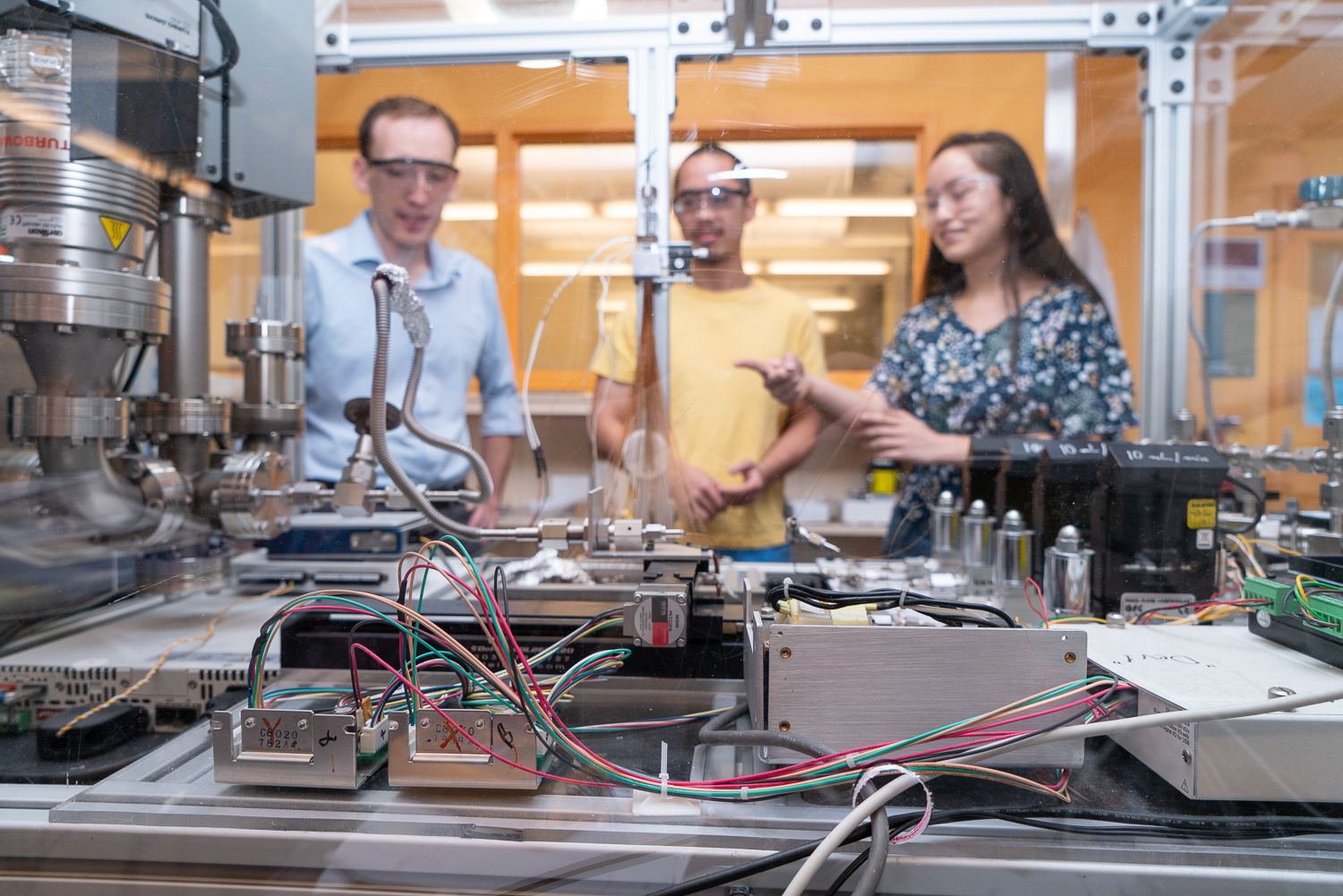
Source: Department of Chemical Engineering, Carnegie Mellon University
Catalysts create change.
Many a middle school science teacher has dripped a few drops of potassium iodide into hydrogen peroxide and watched the delight of their students as a volcano of foam erupted from the container. This experiment is often the way young people first learn about catalysts as something that that can induce a chemical reaction.
But catalysts can make more than foam. As those young people grow into young scientists, they learn that catalysis—the acceleration of a chemical reaction by a catalyst—is a key process in the creation of just about everything. From the plastics that make up our medical equipment, to the gasoline in our cars, to the paint that colors our homes—none of these could exist without catalysts.
Catalysts come in all shapes and sizes, and each one serves a different function. The discovery of new catalysts often means that we are able to create and perfect new materials, which can be used in future products, fuels, and just about everything else. Unfortunately, discovering and optimizing these new catalysts can be a long and difficult process, involving an unruly number of variables. The difficulty of this process is one of the primary barriers to new catalyst discovery.
For this reason, Carnegie Mellon chemical engineers have recently begun to look to other fields for answers. Recently, both the Department of Energy and the National Science Foundation have invested in the unique research that Zachary Ulissi, John Kitchin, and Andrew Gellman are pioneering, which looks into the role that machine learning can play in the discovery of new catalysts. Through the development and implementation of novel machine learning algorithms, the rate at which researchers can discover new, effective catalysts will increase exponentially.
The field of catalysis is embracing machine learning to help solve challenges that have eluded us until now.
Zachary Ulissi, Assistant Professor, Department of Chemical Engineering, Carnegie Mellon University
Optimum alloy composition for catalytic surfaces
Hydrogen fuel cells are powered by catalytic reactions—in particular, by what’s known as an alloy catalyst surface. The efficiency of the fuel cell is dependent on the exact mix of metals that make up that alloy catalyst surface. But finding that perfect mix isn’t easy. That’s why ChemE Professor John Kitchin, with support from the National Science Foundation, has developed a unique machine learning algorithm to rapidly test as many combinations as possible. His findings are detailed in his paper, “Modeling Segregation on AuPd(111) Surfaces with Density Functional Theory and Monte Carlo Simulations,” published in the Journal of Physical Chemistry.
Metal alloys are used as catalysts to produce hydrogen peroxide from hydrogen and oxygen for use as a renewable green oxidant in chemical synthesis. In the case of Kitchin’s research, that alloy is made up of gold (Au) and palladium (Pd). When the palladium reacts with the hydrogen and oxygen in the reactor, it creates hydrogen peroxide, which can be used as an oxidant. Unfortunately, through this reaction, palladium also creates water, which is undesirable for the because it wastes the valuable hydrogen. By alloying gold with the palladium, this secondary reaction can be mitigated, causing the reactor to create more of the desired hydrogen peroxide. But just how well the alloy is able to do this is dependent on the exact ratio of Au to Pd in the catalyst—down to the atom. Checking every possible ratio by hand would take far more time than any group of researchers would be able to spend.
Intermetallics vs. alloys in the quest for efficiency
Alloy catalyst surfaces have a number of applications in chemical engineering—but they are not the only metal catalysts widely used for chemical reactions. Intermetallics are similar to alloys, but instead of the atoms being randomly mixed, intermetallics are created by specifically placing atoms of one metal in a repeating pattern with the atoms of another. Because of their precise atomic composition, intermetallics can be customized specifically to catalyze a particular reaction.
But because atomic placement in intermetallics is so precise, optimizing the arrangement for maximum catalytic effect is an arduous process. Experimentation to develop better intermetallics largely relies on the ‘guess and check’ method. So in order to create a more efficient method, ChemE Assistant Professor Zack Ulissi, along with his collaborators at Penn State, are working to develop a computational tool that uses machine learning to not only model intermetallic configurations and test them for efficiency, but uses the data gathered from these experiments to decide what configurations are more likely to work in the future. The research is supported by a $1.2 million dollar grant from the U.S. Department of Energy.
Experimental tools to confirm machine learning models
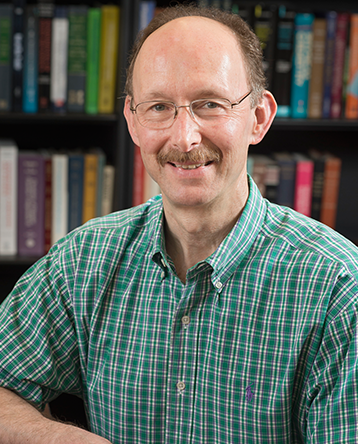
Source: Department of Chemical Engineering
Lord Professor of Chemical Engineering Andrew Gellman
While machine learning is a powerful tool, the ability to experimentally confirm the results of machine learning models is paramount to ensuring their reliability. That’s why Professor Andrew Gellman and his research group have developed experimental methods to complement the machine learning tools developed by Kitchin and Ulissi. The National Science Foundation, through its Designing Materials to Revolutionize and Engineer Our Future (DMREF) initiative, has invested in a team led by Gellman to pioneer brand-new research tools, which can prepare hundreds of alloy compositions simultaneously and concurrently analyze their surfaces.
These tools work by identifying the optimal composition of two or three component alloys, and comparing them to the compositions predicted by machine learning. These component alloys can then be experimentally tested in the lab to confirm that they operate as the machine learning model says they do. Then, once the experiment has corroborated the predictions of the model for several binary and ternary alloys, the optimal compositions of other alloys with different components can be reliably identified on the basis of the machine learning methods alone.
This synergy of theory and experiment underpins a powerful new approach, which will allow for the acceleration of materials development for many applications.
Andrew Gellman, Lord Professor of Chemical Engineering, Department of Chemical Engineering, Carnegie Mellon University
Carnegie Mellon researchers are at the forefront of machine learning for catalysis, and the breadth and depth of this research is always expanding. Students from all over the world come to the department of Chemical Engineering to study this exciting, emerging field. New projects are being funded every day, including a recent ARPA-E grant to support Gellman and Ulissi in studying deep reinforcement learning in catalysis. Thanks to the culture of Advanced Collaboration®, which is deeply native to CMU, ChemE faculty, students, and partner organizations are poised to bring unprecedented change to the field of catalysis discovery.